The Evolution and Challenges of QSAR Modelling in Toxicology – A Closer Look at Recent Advances
- Elena Fioravanzo
- Feb 5
- 3 min read
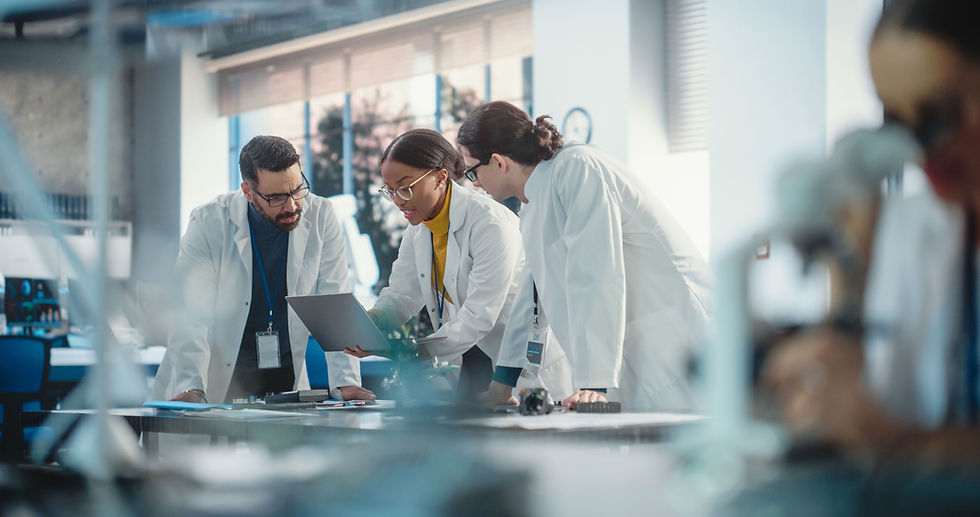
The famous quote by Paul Ehrlich, “To err is human; to really foul things up requires a computer,” humorously encapsulates the challenges that we face when relying on computational models to predict real-world phenomena. In the world of toxicology, the use of Quantitative Structure–Activity Relationship (QSAR) models has become a cornerstone for predicting the biological activity and toxicological effects of chemicals based on their molecular structure. These models are not just academic; they have significant applications in regulatory assessments, including supporting chemical safety evaluations like the EU REACH regulation.
However, as with any powerful tool, QSAR models come with their own set of challenges. This post highlights some of the key insights from a recent scientific paper on QSAR performance, discussing both the lessons learned and the opportunities for improvement.
Training in Computational Toxicology
As we continue to explore these advanced modelling techniques, it’s important to ensure that professionals in the toxicology field are well-equipped to understand and apply them. That’s why we offer specialized training in computational toxicology, covering the principles of QSAR and read-across as part of our NAMs - Use and Application of QSAR and Read-Across course. In this course, we delve into the interpretation of (Q)SAR predictions, as exemplified by this paper.
The Key Concepts Behind QSAR Models
QSAR models seek to establish a relationship between the chemical structure of a compound and its biological or toxicological properties. By doing so, these models can help predict the potential hazards of chemicals without the need for animal testing. Over the years, QSAR models have evolved, moving from simple linear regression methods in the 1960s to more sophisticated multivariate, non-linear models utilizing machine learning algorithms such as deep learning and random forests. Despite their success in many areas, predicting toxicity is inherently complex and varies depending on the chemical space and endpoint being modelled.
A Focus on the Second Ames/QSAR International Challenge
One of the most recent and notable exercises in QSAR modeling for toxicity prediction is the Second Ames/QSAR International Challenge Project, which assessed the ability of models to predict mutagenicity in a dataset of approximately 1,600 chemicals. This study involved over 50 different models created by 21 developers from across the globe. Surprisingly, the results highlighted a stark range in model performance, with balanced accuracy scores ranging from 49.6% to 78.5%.
The paper points to several lessons learned from this project. For example, the poor performance of a deep learning model from Liverpool John Moores University (LJMU) emphasized the importance of quality data, appropriate descriptor selection, and proper modelling approaches. This highlights the need for a clear definition of the problem at hand and a nuanced understanding of the chemical data involved.
Key Takeaways: Improving QSAR Models
The paper emphasizes several areas where QSAR models need to evolve for better accuracy and utility in toxicology:
Data Quality and Relevance: High-quality, relevant datasets are essential for training predictive models. This is particularly true for deep learning models, which require large datasets to perform well. For smaller datasets, techniques like data augmentation and transfer learning can help improve model performance.
Model Interpretability: Complex models, especially those utilizing machine learning, often behave like "black boxes," making it difficult to understand how predictions are made. The paper suggests methods like SHAP (SHapley Additive exPlanations) to help interpret and explain the inner workings of models.
Federated Learning: A promising new approach in QSAR model development is federated learning (FL), which allows for collaborative model development without sharing private datasets. This decentralized approach could lead to better, more robust models while respecting data privacy.
Uncertainty Quantification: Models must not only predict toxicity but also communicate the uncertainty of their predictions. Probabilistic models, such as Bayesian networks, can help address this issue by providing a measure of confidence alongside the prediction.
FAIR Principles: In order for QSAR models to be truly useful, they must adhere to the FAIR principles: Findability, Accessibility, Interoperability, and Reusability. This ensures that models and their data can be easily accessed, reproduced, and applied by others in the field.
The paper is open access, so you can read the full study here.
Conclusion
QSAR models have made significant strides over the past several decades, but there is still room for improvement. With ongoing advancements in data science and machine learning, the future of QSAR modelling looks bright. By addressing the challenges discussed in the recent paper, such as data quality, model interpretability, and uncertainty quantification, we can improve the predictive power of these models and their application in regulatory and safety assessments.
Comments