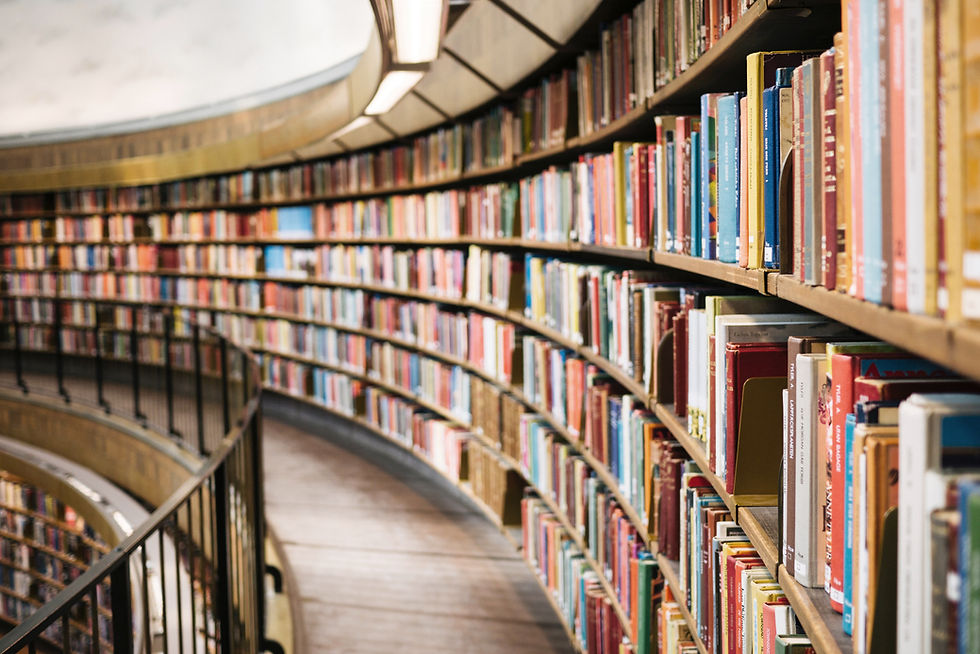
As computational toxicology advances, its role in predicting chemical toxicity is becoming essential in contemporary safety evaluations. Nonetheless, similar to any scientific pursuit, computational methods are subject to uncertainties. A recent paper titled "A Framework for Categorizing Sources of Uncertainty in In Silico Toxicology Methods: Considerations for Chemical Toxicity Predictions", Achar, J.; Firman, J. W.; Cronin, M. T. D.; Öberg, G., provides a structured way to understand and address these uncertainties.
Why This Paper is Important
For those venturing into computational toxicology, appreciating the sources and impacts of uncertainty is essential. This paper offers a comprehensive framework for identifying and categorizing uncertainties in in silico methods. It emphasizes the importance of transparency in model development and results interpretation, fostering trust in computational predictions.
Key takeaways include:
Clear Categorization of Uncertainty: The paper delineates uncertainty into identifiable categories, such as model structure, data quality, and extrapolation challenges.
Practical Considerations for Toxicologists: It bridges the gap between theoretical frameworks and practical applications, making it a valuable resource for understanding the reliability of computational predictions.
Implications for Regulatory Contexts: With increasing reliance on computational models in regulatory settings, understanding uncertainty ensures that models are used appropriately, enhancing confidence in non-animal methods.
Case Study Application: Demonstrating the framework’s utility, the authors evaluate QSAR-based toxicity predictions for chemical safety.
Who Should Read This?
If you are a toxicologist seeking to integrate computational tools into your work, or if you aim to refine your understanding of model predictions, this paper is an excellent starting point. It provides actionable insights for evaluating the robustness of in silico tools and leveraging them effectively in your research or regulatory submissions.
Achar, J.; Firman, J. W.; Cronin, M. T. D.; Öberg, G. A Framework for Categorizing Sources of Uncertainty in in Silico Toxicology Methods: Considerations for Chemical Toxicity Predictions. Regulatory Toxicology and Pharmacology 2024, 154, 105737. https://doi.org/10.1016/j.yrtph.2024.105737.
Explore the full paper here.
For those looking to deepen their understanding of in silico NAMs, I highly recommend checking out our dedicated training course NAMs - Use and application of QSAR and read-across course, which provides valuable insights that complement the discussion in this post.
Comments